1. Why AI Agents Are the New Currency of Power
What if your company, or your entire nation, possessed the ability to print productivity by harnessing intelligence at scale?
This concept gained concrete form with the announcement of an unimaginable $500 billion investment in artificial intelligence infrastructure, which excludes the additional €331 billion independently committed by industry titans like Meta, Microsoft, Amazon, xAI, and Apple. This agreement, spearheaded by President Trump, involves a partnership between the CEOs of OpenAI (Sam Altman), SoftBank (Masayoshi Son), and Oracle (Larry Ellison). Their joint venture, named “Stargate”, aims to build “the physical and virtual infrastructure to power the next generation of advancements in AI,” creating “colossal data centers” across the United States, promising to yield over 100,000 jobs.
France and Europe, not to be outdone, responded swiftly. At the AI Action Summit in Paris in February 2025, French President Emmanuel Macron announced a commitment of €109 billion in AI projects for France alone, highlighting a significant moment for European AI ambition. This was followed by European Commission President Ursula Von Der Leyen’s launch of InvestAI, an initiative to mobilize a staggering €200 billion for investment in AI across Europe, including a specific €20 billion fund for “AI gigafactories.” These massive investments, on both sides of the Atlantic, show a clear objective. The highest level of commitment reflects understanding. This fact translates into one reality: being a civilization left behind is simply out of the question.
But the stakes in this global AI game are constantly rising. If the US and Europe thought they were holding strong hands, China, arguably the most mature AI nation, has just raised the pot. China is setting up a national venture capital guidance fund of 1 trillion yuan (approximately €126.7 billion), as announced by the National Development and Reform Commission (NDRC) on March 6, 2025. This fund aims to nurture strategic emerging industries and futuristic technologies, a clear signal that China intends to further solidify its position in the AI race, focusing among others, on boosting its chip industry.
The implicit “call” to the other players is clear: Are you in, or are you out?
Therefore, my first question isn’t issued from a sci-fi movie; it’s not some fantastical tale ripped from the green and black screen of The Matrix, where programs possessed purpose, life, and a face.
This is about proactively avoiding the Kodak moment within our respective industries.
This is about your nation avoiding the declining slope of Ray Dalio’s Big Cycle, where clinging to outdated models in the face of transformative technology is a path toward obsolescence.
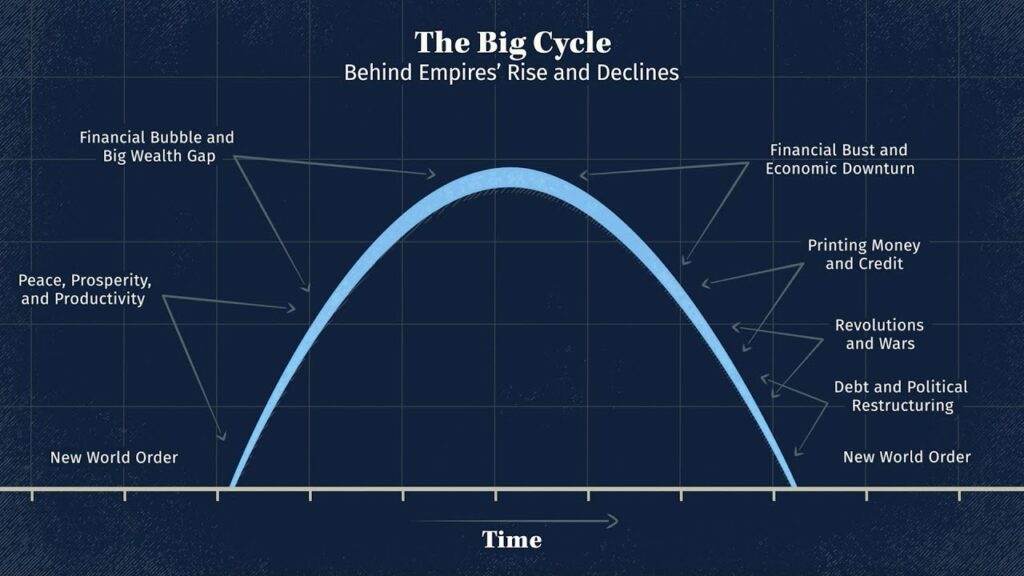
This is the endgame: AI Agents are not just changing today—they are architecting the future of nations.
2. Architecting Sovereignty: How Nations Are Industrializing Intelligence
In November 2024, I had the privilege of delivering a second course on Digital Sovereignty, focused on Artificial Intelligence, at the University of Luxembourg, thanks to Roger Tafotie. I emphasized that the current shift toward advanced AI, especially Generative AI, represents a paradigm shift unlike before. Why? Because, for the first time in history, humanity has gained access to the near-infinite scalability of human-level intelligence. Coupled with the rapid advancements in robotics, this same scalability is now within reach for physical jobs.
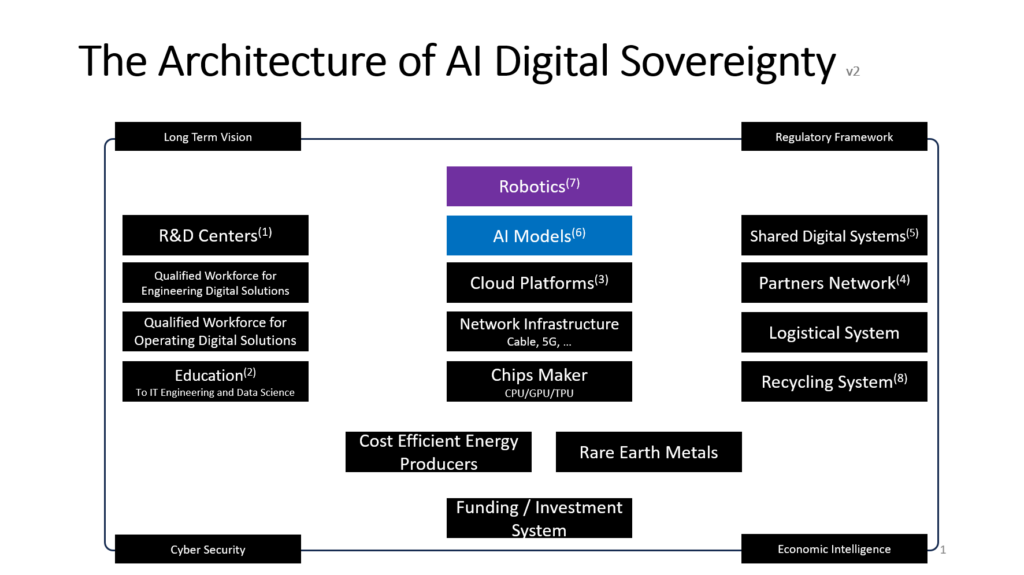
Digital Sovereignty in the age of AI is a battle for access to the industrialization of productivity. Consider the architecture of AI Digital Sovereignty as a scaffolding built of core capabilities, much like interconnected pillars supporting a grand structure:
- Cloud Computing: The foundational infrastructure, the bedrock upon which all AI operations rest.
- AI Foundation Model Training: This is where the raw intelligence is refined, like a rigorous academy shaping the minds of future digital workers.
- Talent Pools: The irreplaceable human capital, the architects, engineers, data scientists, and strategists who drive innovation. These are the skilled individuals, the master craftspeople, forging the tools and directing the symphony of progress.
- Chip Manufacturing: The ability to produce advanced CPU, GPU, and specially designed AI chips, like TPU and LPU, guarantees independence.
- Systems of Funding and Investments: The ability to finance a long-term, consistent, and high-level commitment toward AI capabilities.
If you need a tangible example of how critical resources like rare earth metals and cheap energy are to this race, look no further than President Donald Trump’s negotiations with Volodymyr Zelenskyy. The proposed deal? $500 billion in profits, centered on Ukraine’s rare earth metals and energy reserves. Let’s not forget: 70% of U.S. rare earth imports currently come from China. Control over these resources is the lifeblood of AI infrastructure.
It’s not merely about isolated components but how these elements interconnect and reinforce each other. This interconnectedness is not accidental; it’s the key to true sovereignty – the ability to use AI and control its creation, deployment, and evolution. It’s about building a self-sustaining ecosystem, a virtuous cycle where each element strengthens the others.
Europe initially lagged, but the competition has only just begun. The geopolitical landscape will be a major, unmastered factor.
The current market “game” consists of finding the critical mass between the hundreds of billions invested in R&D, the availability of “synthetic intelligence”, and unlocking a new era of sustainable growth. The race to discover the philosophical stone—to transform matter (transistors and electricity) into gold (mind)— and to achieve AGI and then ASI is on.
Sam Altman knows it; his strategy is “Usain Bolt” speed: the competition cannot keep up if you move at a very fast pace with AI product innovation. Larry Page and Elon Musk intuitively grasped this first. OpenAI was not only created to bring “open artificial intelligence” to each and every human, but also serves as a deliberate counterweight to Google DeepMind. Now, Sundar Pichai feels the urgency to regain leadership in this space, particularly now that Google Search—the golden goose — is threatened by emerging “Deep Search” challengers such as Perplexity, ChatGPT, and Grok.
As of March 2025, HuggingFace, the premier open-source AI model repository, has more than 194,001 text generation models (+24.1% since November 2024) within a total of 1,494,540 AI models (+56.8 % since November 2024). Even though these numbers include different versions of the same base models, think of them as distinct blocks of intelligence. We are already in an era of abundance. Anyone possessing the necessary skills and computational resources can now build intelligent systems from these models. In short, the raw materials for a revolution are available today.
The stage is set: The convergence of human-level intelligence scalability and robotics marks a profound moment in technological history, paving the way for a new era of productivity.
3. The Revolution of the Agentic Enterprise
In October 2024, during the Atlassian Worldwide conference Team 24 in Barcelona, I had the privilege of seeing their integrated “System of Work” firsthand.
Arsène Wenger, former Football Manager of Arsenal FC and current FIFA’s Chief of Global Football development, was invited to share his life experiences in a fireside chat. It was a true blessing from an ultimate expert in team building and the power of consistency.
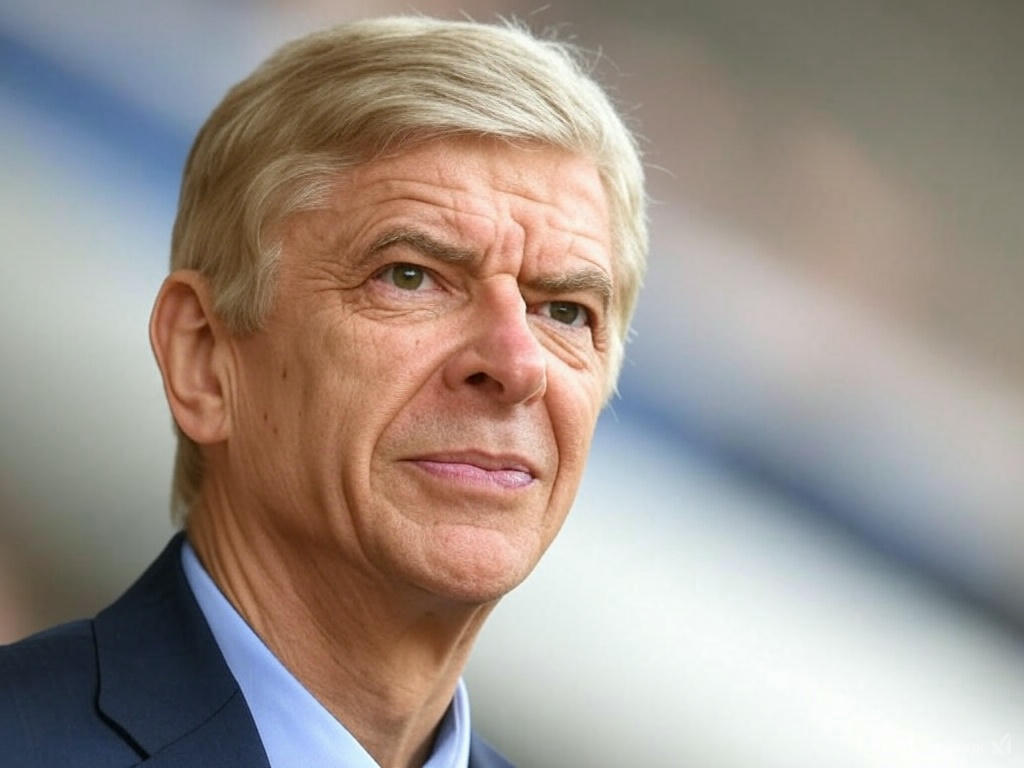
Mr. Wenger articulated that the conditions and framework for achieving champion-level performances rely on a progressive and incremental journey. While talent can confer a slight edge, that edge remains marginal in the realm of performance. The key differentiator resides in consistent effort, with a resolute commitment to surpassing established thresholds. Regularly implementing extra work and consistently reaching your capacity is what separates a champion from the rest.
Thus, Atlassian pushed their boundaries, unveiling Rovo AI, an agentic platform native to their environment. Rovo is positioned at the core of the “System of Work,” bridging knowledge management with Confluence and workflow mastery with Jira. To my surprise, Atlassian announced they have 500 active Agents! This is a real-world example of printing productivity by deploying purpose-driven digital workers to the existing platform.
But the true brilliance lies in making this digital factory available to their customers. This type of technology should be on every CEO and CIO’s roadmap. How you integrate this capability into your business and technology strategy is the only variable – the fundamental need for it is not.
The Agentic Enterprise is the cornerstone of this change: creating autonomous computer programs (agents) that can handle tasks with a broad range of language-based intelligence.
We’ve transitioned from task-focused programming to goal-driven prompted actions. Programming still has a role to play as it guarantees perfect execution, but the cognitive capabilities of large language reasoning models like GPT O3 and Deepseek R1 lift all its limitations. Moreover, in IT, development is now about generated code. While engineering itself does not necessarily become easier – you still need to care about the algorithm, the data structure, and the sequence of your tasks – the programming part of the process is drastically simplified.
After years of prompt engineering since the GPT3 beta release at the end of 2020, I concluded that prompt engineering is not a job per se but a critical skill.
The Agentic Enterprise is not a distant dream but a present reality, fundamentally changing how organizations construct and scale their work.
4. Klarna’s AI-Powered Customer Service Revolution: How AI Assumes 2/3 of the Workload
In February 2024, the Swedish fintech Klarna announced that their AI contact center agent was handling two-thirds of their customer service chats, performing the equivalent work of 700 full-time human agents. It operates across 23 markets, speaks 35 languages, and provides 24/7 availability.
With full speech and listening capabilities now available in models like Gemini 2 Realtime Multimodal Live API, OpenAI Realtime API, or VAPI, the automation opportunities are virtually limitless.
What made this rapid advancement possible, suddenly?
Technically, the emergence of multimodality in AI models, robust APIs, and the decisive capability of Function Calling form the foundation. But more importantly, this transformation is primarily a business vision; it lies in adopting transformative technology as the main driving power, and using innovation as your key distinguishing factor, just as with Google and OpenAI. When this approach acts as the central nervous system in business strategy, then, the adoption is not perceived as a fundamental disruption but, rather, a gradual and consistent reinforcement.
The crucial capability here is Function Calling, which allows AI models to tap into skills and data beyond their inherent capabilities. Think of getting the current time or converting a price from Euros to Swiss Francs using a live exchange rate – things the model can’t do on its own. In a nutshell, Function Calling lets the AI interact directly with APIs. It’s like giving the AI a set of specialized tools or instantly teaching it new skills.
APIs are the foundation for the intelligence relevance of AI Agents and their ability to use existing and new features based on user intent. In contrast to prior generations of chatbots, which needed explicit intent definitions for each conversation flow, LLMs now provide the intelligence and the knowledge “out of the box.”. To further understand the fundamental importance of APIs for any modern business, I invite you to read my article or listen to my podcast episode titled “Why APIs are fundamental to your business“.
This has led to the rise of startups like Bland.ai that offer products like Call Agent as a service. You can programmatically automate an agent to respond over the phone, even customizing its voice and conversation style – effectively creating your own C3PO.
ElevenLabs, the AI voice company that I use for my podcast, has also launched a digital factory for voice-enabled agents.
Then, December 18 2024, OpenAI introduced Parloa, a dedicated Call Center Agent Factory. This platform represents the first of its kind, specializing in digital workers for a specific industry vertical.
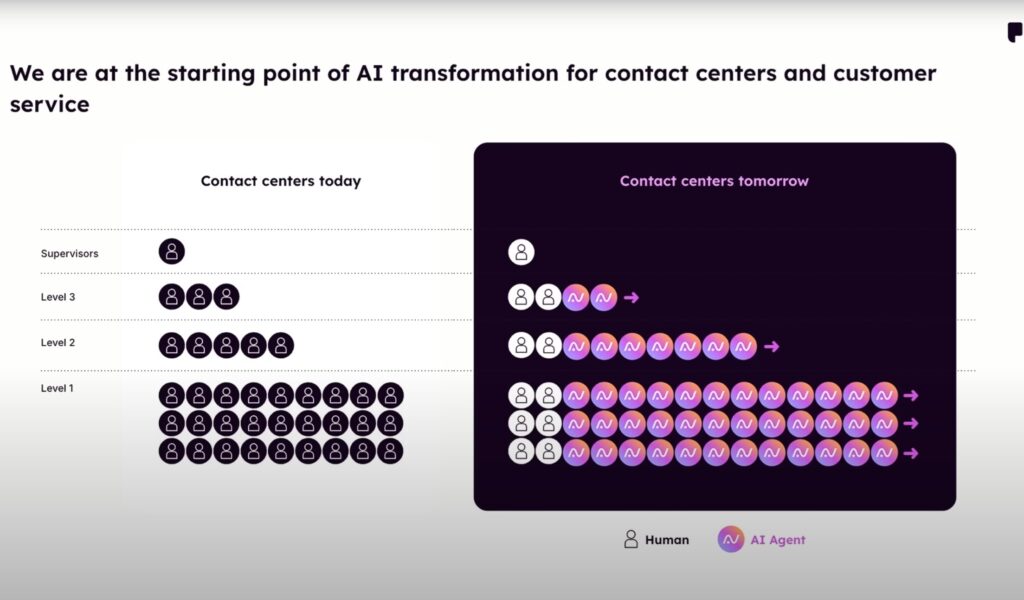
The promise? To transform every call center officer into an AI Agent Team Leader. As a “Chief of AI Staff”, your objective will be to manage your agents efficiently, handling the flow of demands, intervening only when necessary, and reserving human-to-human interactions for exceptional client experiences or complex issues.
The revolution in customer service is already here, driven by new AI-based call center solutions. This is a sneak peek of the AI-driven future.
5. The Building Blocks: AI Development Platforms
To establish a clear vision for an AI strategy and augment my CTO practice, I meticulously tested several AI technologies. My goal is to validate the technological maturity empirically, assert the productivity gains, and, more importantly, define the optimal AI Engineering stack and workflow. These findings have been documented within my AI Strategy Map, a dynamic instrument of vision. As a result, my day-to-day habits have completely changed and reflect my emergence as a full-time “AI native”. My engineering practice is reborn in the “Age of Augmentation“.
I changed my stack to Cursor for IT development, V0.dev for design prototyping, and ChatGPT o3 for brainstorming and review. The results achieved so far are highly enlightening and transformational.
Thus, engineering teams’ next quantum leap is represented by the arrival of Agentic IDEs, facilitating an agent-assisted development experience. The developer can seamlessly install the IDE, create or import a project, input a prompt describing a feature, and observe a series of iterative loops leading to the complete implementation of the task. The feature implementation is successful in 75% of cases. In the remaining 25%, the developer issues a corrective prompt to secure 100% implementation, indicating a need to supervise such technology when used as an independent digital worker.
Leading today’s innovative Agentic IDE market are:
- Cursor AI: https://www.cursor.com/
- Windsurf AI: https://codeium.com/windsurf
Then, the landscape of mature AI frameworks gives companies a great array of enterprise-ready solutions. Automated agent technologies, specifically, have emerged as critical tools:
- OpenAI Agents SDK (previously Swarm) and Agent development tools
- LangChain Agents: https://www.langchain.com/agents
- CrewAI: https://www.crewai.com/
- Pydantic Agents: https://ai.pydantic.dev/agents/
- Microsoft Autogen Agent Framework: https://microsoft.github.io/autogen/
- ElevenLabs Conversational AI: https://elevenlabs.io/conversational-ai
Finally, DEVaaS platforms are completely redefining the approach to IT delivery :
- Bolt.New: https://bolt.new/
- Replit AI: https://replit.com/ai
These technologies allow you to build applications from the ground up that are fully operational right from the start, without any additional setup time. Yet, it is not only about developing an application from scratch and then gradually adding features by using prompts as your instruction; it’s also about application hosting. These solutions now offer a complete DevOps and Fullstack experience.
Although they currently yield simple to moderately complex applications, and may not be entirely mature, the technologies are demonstrably improving at rapid paces. It is only a matter of weeks (not months) before seeing full system decomposition and interconnectedness that reaches corporate standards.
This is exactly what the corporate environment has been waiting for.
How will this impact the IT organization? It comes down to these three outcomes:
First, for companies where the IT organization is a strategic differentiator, the internal IT workforce will increase productivity by delegating development tasks directly to internal AI Agents. Sovereign infrastructure is most likely required in heavily regulated or secretive industries.
Second, companies where IT is a primary activity, but not a core organizational driver can outsource the IT development to specialized companies who also make use of Agentic development IDEs or platforms.
Finally, an option for outsourcing includes the reconfiguration of jobs and empowering base knowledge workers – individuals with no official IT background – to IT positions through AI training. This would be the full return of the Citizen Developer vision, previously promised by Low Code/ No Code trends.
Innovation within the AI domain occurs several times per day, thus requiring a proactive mindset to remain updated technically which leads into actionable technology perspectives.
6. The Power of Uni-Teams: The Daily Collaboration Between Man and Machine
What does it feel like to lead your own AI team?
From experience, it make you feels like Jarod, from the TV show “The Pretender“, a true one-man band capable of handling multiple roles.
Think of it this way, you are now capable of:
- Writing code like a developer
- Creating comprehensive documentation like a technical writer
- Offering intricate explanations like an analyst
- Establishing policy frameworks like a compliance officer
- Originating engaging content like a content writerData storytelling like a data analyst
- Data storytelling like a data analyst
- Building stunning presentations like a graphic designer
From my experience, reaching mastery in any discipline often reveals an observable truth within the corporate world: a significant portion of our tasks are inherently repetitive. The added value is not in running through the same scaffolding process many, many times, if you are no longer learning (except perhaps to reinforce previous knowledge). The real value is in the outcome. If I can speed up the process to focus on learning new domain knowledge, multiplying experiences, and spending time with my human colleagues, it is a win-win.
My “Relax Publication Style“, an guided practice for anyone starting in content creation, has evolved into a more productive and enjoyable method – combining iterative feedback cycles from human/AI, to leverage strategic insights via structured ideas, ongoing AI-reviews, personal updates and enriched content using human-curation combined with in-depth AI search tools. I will explore this process in a future article.
One of the most surprising shifts in my workflow has been the emergence of “background processing” orchestrated by the AI Agent itself. It’s a newfound ability to reclaim fragments of time, little pockets of productivity that were previously lost. It unfolds something like this:
- The Prompt: I issue a clear directive, the starting point for the AI’s work.
- The Delegation: I offload the task to the AI, entrusting it to this silent, tireless digital worker.
- The Productivity Surge: I’m suddenly free. My capacity is expanded, almost as my productivity is nearly doubled. I can tackle other projects, collaborate with another AI agent, or even (and I’m completely serious) indulge in a bit of gaming.
- The Harvest: I gather the results, reaping the rewards of the AI’s efforts. Sometimes, it’s spot-on; other times, a refining prompt is needed.
In my opinion, this is a deep redefinition of “teamwork.” It’s no longer just about human collaboration; it’s about orchestrating a symphony of human and artificial intelligence. This is the definition of “Ubiquity.” I work anytime and truly anywhere – during my commute, in a waiting room, even while strolling through the park (thanks to the marvel of voice-to-text). It’s a constant state of potential productivity, a blurring of the lines between work and, well… everything else.
The next stage begins with gaining awareness and using AI. From there, it progresses to actively building your AI teams. The goals of a collaboration with agents, for instance, for a product designer or a system architect, would be to actually create an AI team so that a human product designer becomes, in fact, the team leader of their AI agents. Let’s see how and why.
The rise of AI teammates promises greater productivity and a fundamental shift in the way we approach problem-solving and work.
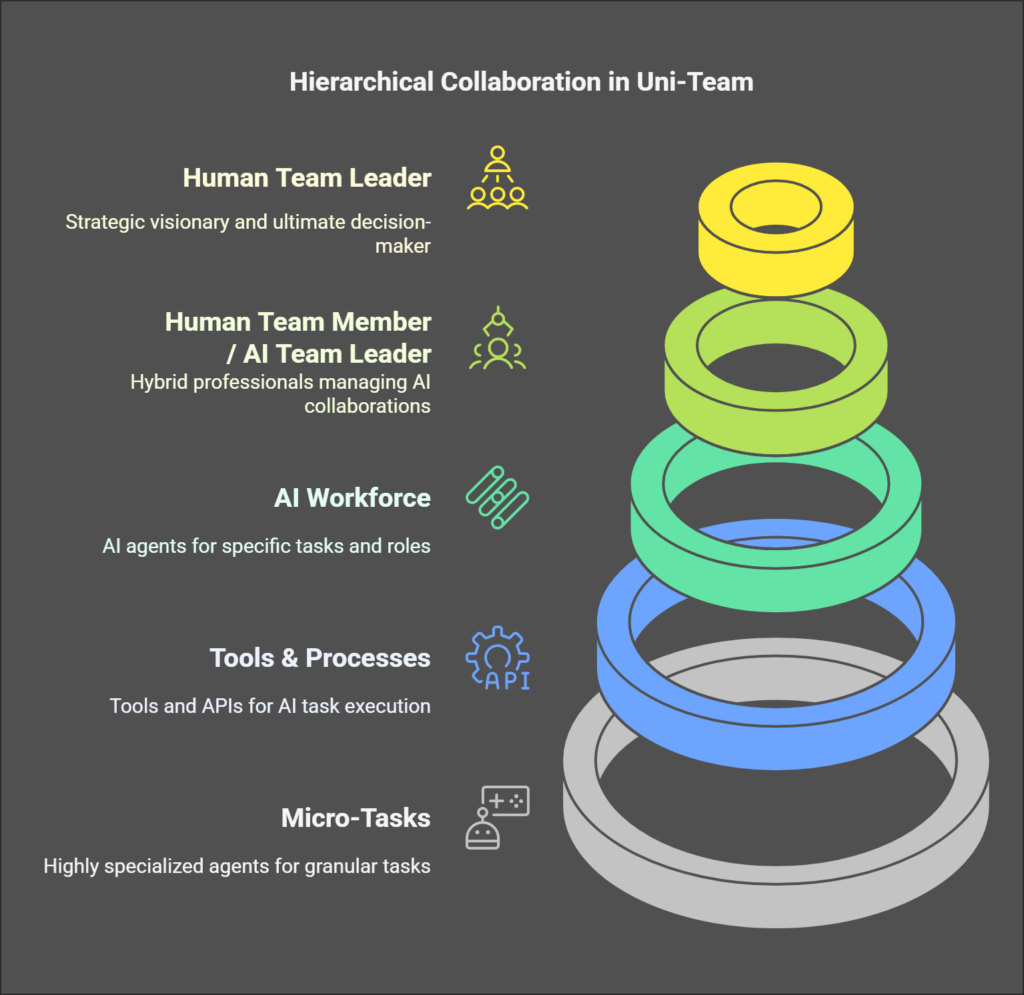
7. Evolving as a Knowledge Worker in the Age of AI
How can a worker adapt to the capabilities expansion of printing productivity?
It starts by understanding the current capabilities of AI; exploring them through training or by testing systems, and see how they can be applied in your own workflow. Specifically, with your existing tasks, determine which ones can be delegated to AI. Even though, at the moment, it’s more about a human prompting and the AI executing a small, specific task. Progressively, these tasks will be chained, increasing the complexity to the level of higher hierarchical tasks. This integration of increasingly complex tasks constitutes the purpose of agents – to have a certain set of skills handled by AI.
For functional roles such as product designers or business analysts, there is an opportunity to transition toward a product focus, to understanding customer journeys, psychology, behaviors, needs, and emotions. This can result in an experience (UX) driven approach, where the satisfaction of fulfilling needs and solving problems is paramount while leveraging data insights to enhance the customer’s experience.
Indeed, this technology is already showcasing its potential to bring us together. Within my organization, my colleagues are openly sharing a feeling of relief at how Generative AI empowers them by significantly reducing the workload of some specifically tedious tasks from days to mere minutes. But it is not all about saving time: the key progress that made me really moved, is that now, freed from a few tedious operations, they have now the capacity to explore their current struggles, identify past pain points, and articulate new business requirements. Additionally, it is the “thank you”, that directly acknowledges my teams’ efforts in bringing this new means to reclaim precious time and comfort. What is even far more compelling, and very inspirational from my point of view, is this ability to formulate and then resolve this new set of challenges using the capabilities of this recent AI ecosystem. Witnessing this emerging transformation gives me tangible joy and concrete hope for our collaborative future.
So, the key observation is that it’s up to early adopters and leaders to drive this change. They need to build a culture where people aren’t afraid to reimagine their jobs around AI, to learn how to use these tools effectively, and to keep learning as the technology evolves. The time to strategize how AI reshapes internal processes to master inevitable industry restructuration has arrived while simultaneously positioning your organization as a demonstrative leader for others to follow. To build that next-generation workforce, you need tools and specific actionables strategies; what are the core components to your next plan?
Ultimately, this era of augmentation is a strategic opportunity – one that requires everyone involved, including users and top executives, to actively foster continuous understanding, ongoing discovery, and strategic adaptation, thus contributing at multiple levels to building high-performing teams.
Disrupt Yourself, Now.
8. The Digital Worker Factory: A Practical Example in Banking
Let’s consider a practical example. Imagine delivering a project to create an innovative online platform that sells a new class of dynamic loans—loans with rates that vary based on market conditions and the borrower’s repayment capacity. This platform would be fully online, SaaS-based, and built as a marketplace where individuals can lend and borrow, with a bank acting as a guarantor. That is the start of our story. Now it’s about delivering this product.
What if you only needed a Loan Product Manager, a System Architect, and a team of agents to bring this digital platform to life?
Here’s how the workflow looks.
- As the product manager, you specify the feature set and map the customer journey from the borrower’s perspective. You define the various personas – a lender, a borrower, a bank, and even a regulator.
- The system architect then set up the technical specifications for the IT applications and LLMs, covering deployment to the cloud, integrations such as APIs, data streams, and more.
- You initiate the iterative loop by defining a feature. The AI Agent then plans and generates the code, after which you test the feature. Based on your feedback, the Agent troubleshoots and corrects the program accordingly. This loop continues iteratively until the platform fully takes shape. In this workflow, the product isn’t merely coded—it’s molded. The prompt itself becomes the new code.
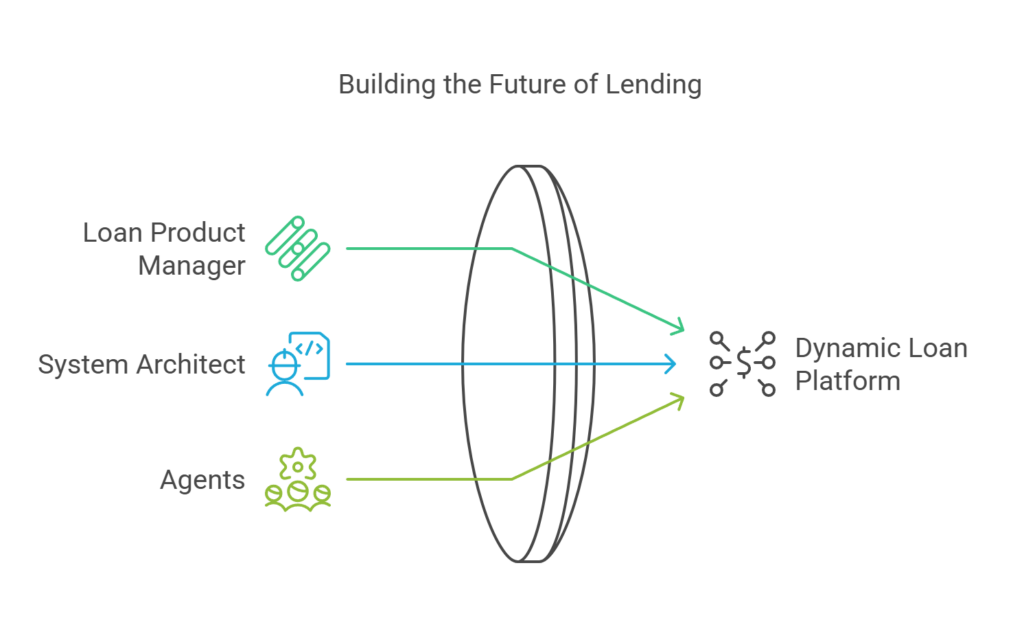
With a clear vision and the right framework, the path to production is not as complicated as it once was.
The Loan Expert Augmented: AI in Action
Consider the Loan Product Manager. They use AI to simulate loan profitability, examining various customer types and market variations. But, as importantly, they use AI to refine pitches, sales materials, and regulatory documentation. This results in streamlining compliance and ensures alignment with the existing framework.
Generative AI is also used to revise internal and external processes. The templates for product sheets are optimized and iteratively improved. Marketing materials, such as a webpage explaining the product, equally take advantage of Artificial Intelligence to reach the best clarity and impact.
Finally, personalized communication with a customer per specific client also relies upon Generative AI automation and data contextualization. If a customer needs a loan for a car or other tangible asset, the communication is perfectly tailored to the specific context.
This is personal banking at scale.
The focus is on the active role workers play in orchestrating, managing, and continuously evolving the AI systems they rely on in their daily work.
Hence, we’re effectively printing productivity now – a rare paradigm shift. Every professional needs to be proactive to seize this opportunity, not just react to it. Start by exploring AI tools relevant to your field, experiment with their capabilities, and consider how they can be integrated into your daily workflows. Whether you are a software engineer, product designer, or loan expert, the time to adapt is now.
Bear with me: the way you’ve operated up to this point—with data entry in applications, scrupulously following procedures, and writing lengthy reports in document processing software— is now directly challenged by individuals adopting the “automatician” mindset, evolving their skills from basic Excel macros into sophisticated, full-fledged applications. But remember: The future is not something you passively face. This world is your design, using available new technologies along with your pragmatic actions
9. Integrating AI Engineering in your System of Delivery, The Two Paths Forward: New vs Existing Systems
Now that Generative AI has entered your work and that you are integrating all the different aspects of the digital workers – either through pilot projects or all other internal activities – this awareness converges towards one strategic decision.
This decisive decision leads to two clear paths: either build a completely new application and workflow designed from the ground up around AI-augmented technologies or modernize existing complex systems to align with current AI-powered delivery.
Your choice needs solid considerations, even though both outcomes must lean toward a similar goal: a fully modern and flexible digital workforce, printed from your Enterprise Agent Factory. Thus, ensure you keep a strategic direction of impact toward a significantly better system.
Path 1, Building from Scratch: The AI Native Approach
The first and straightforward path involves building completely new systems. Here, the software specifications are essentially the prompts within a prompt flow. Think of this prompt flow as the blueprint for the code, all directly created within an Agentic IT delivery stack. The advantage of this approach is that the entire system is designed from the ground up to work seamlessly with AI agents. It’s like building a house from scratch with an AAA energy pass and all the home automation technologies included.
The prerequisite of this stage is to constitute a core team of pioneers that went successfully into production with at least one product used by internal or external clients. In the process, they successfully earned their battle scars, gained experience, selected their foundation technologies, established architectural patterns, and built a list of dos and don’ts, which ultimately will turn into AI Engineering guidelines and best practices.
Next – and this decisive break from the old system is non-negotiable – this group must devise a brand new method of work: building its strategic and actionable steps in all operational components that allow AI to be present from day one and without the limitation of legacy infrastructure that no longer fits. Because all this experience now results in a unique point of reference, a key ”baseline” from that point you can start designing a process to enable the full transformation from the current to future operations.”
Path 2, Evolving From Within: Growing AI Integration in the Existing Enterprise Application Landscape
The second path involves evolving existing systems. This is a more intricate process as it requires navigating the complexities of the current infrastructure. Engineers accustomed to the predictability and consistency of traditional coding methods now need to adapt to the probabilistic nature of AI-driven processes. They must deal with the fact that AI outputs, while powerful, can not always be exactly the same.
Initially, this can be unsettling because it disrupts your established practices, but with tools such as Cursor or GitHub Copilot, you can quickly become accustomed to this new approach.
This shift requires that software engineers move from the specific syntax of languages like Python or TypeScript to communicate in everyday language with the AI, bringing skills that were previously specific to them into the reach of other knowledge workers. Furthermore, it is not easy to introduce powerful LLMs in a piece of software that has an established code structure, architecture, and history. It’s like renovating an old house – you are forced to work with existing structures while introducing AI elements. This requires a deep understanding of the current code and the implications of architectural choices, such as why you would use Event Streaming instead of Synchronous Communication or a Neo4J (graph database) instead of PostgreSQL (relational database) for a specific task.
Accessing and integrating with legacy systems adds another layer of friction because the code is outdated or uses a proprietary language. While AI facilitates code and data migration, the increased efficiency of AI-native platforms often makes rewriting applications from scratch the most optimal strategy.
In summary, creating AI-native applications from scratch is easier, with an incredible speed of development, but it implies a bold decision. Transitioning an existing application is more difficult, as it has inherent architectural, data, or technological constraints, but it is the most accessible path for many companies.
The increasing power of LLMs to handle ubiquitous tasks that were previously exclusively human tasks implies a compression of tasks and skills within an AI. This shift moves some work regarding coordination, data management, and explanatory work from humans to machines. For human professionals, this will result in the reduction of these types of tasks, freeing them to focus on higher-level tasks.
The duality of paths ahead is a call for a pragmatic approach to transition; it’s about moving forward without disrupting too much of the familiar workplace.
10. The Metamorphosis: From Data Factories to Digital Workforce Factories
[Picture: A symbolic image representing a butterfly emerging from a chrysalis]
Today, we are gradually fully exploiting the potential of Generative AI, with text being the medium to translate, think, plan, and create. These capabilities are expanding to media of all kinds – audio, music, 3D models, and video. Consider what Kling AI, RunwayML, Hailuo, and OpenAI Sora are capable of; it is just the beginning and building blocks of what is possible.
These capabilities, originally for individual tasks, are now transforming entire industries – architecture, finance, health care, construction, and even space exploration, to name just a few.
If you can automate aspects of your life, you can automate parts of your work. You can now dictate entire workflows, methods, and habits. You can delegate. What’s the next stage?
So far, we have created automatons, programs designed to execute predefined tasks to fulfill a part of a value chain. These are digital factories comparable to factories in the physical world that have built computers, cars, and robots. And now, if you combine factories and robotics with software AI, the result is the ultimate idea: the digital worker.
The key is that it is no longer just about using or building existing programs but more about building specific agents. These agents represent specialized versions of the human worker and include roles such as software engineers, content creators, industrial designers, customer service providers, and sales managers. Digital workers have no limits in scaling their actions to multiple clients and languages at the same time.
The new paradigm consists of creating a new workforce. We used to construct data factories with IT systems, and now, with Generative AI, we are building Digital Workforce Factories. A Foundation Model is the digital worker’s brain. Prompts are defining their job function within the enterprise. API and Streams are their nervous system and limbs to act upon the real world and use existing code from legacy systems.
The extensive time once required to cultivate skilled human expertise—spanning roughly eighteen years in formal education, followed by years of specialization, and reinforced through real, tangible work applications— is now radically compressed due to the capacity of LLM technology. By which, as I detailed previously in “Navigating the Future with Generative AI: Part 1, Digital Augmentation“, it all highlights our mastery in having compressed centuries of structured knowledge: from methodical research, systematic problem-solving frameworks, and many and countless cycles of innovation process from implementation best practices. Still, key expertise now resides in both the method and application. New competencies should prioritize AI foundational model mastery, the value of highly skilled fine-tuning methods for specific domain applications, and how to leverage creative prompts to build a tangible output from such systems even with unexpected new scenarios.
It is paramount to fully grasp that what we experience now with AI transformation is more than just a set of groundbreaking techniques. It reveals a new structure—for better and for worse—impacting both knowledge workers assisted by AI agents and manual workers augmented by robotics. But remember, ‘and’ is more powerful than ‘or’: it is precisely the combination and convergence of these roles — human and digital working together — that creates true scalability and transformative potential.
The real path forward isn’t merely augmentation—it’s about fostering a genuinely hybrid model, emerging naturally from a chrysalis stage into a mature form. This new human capability is seamlessly amplified by digital extensions and built upon robust foundations, meticulously refined over time. Moreover, humans are destined to master Contextual Computing, where intuitive interactions with a smart environment—through voice, gesture, and even beyond—become second nature. This isn’t about replacing humans; it’s about elevating them to orchestrate a richer, more integrated digital reality.
Perhaps artificial intelligence is the philosopher’s stone—the alchemist’s ultimate ambition—transmuting the lead of raw data into the gold of actionable intelligence, shaping our environment, one prompt at a time.
Dear fearless Doers, the future is yours.